Reza Sadri, the director of the new AI Bootcamp in the Division of Engineering and Applied Science (EAS), comes to Caltech with a mission: to empower scientists and engineers with a deeper understanding of artificial intelligence (AI) and its applications within research. Drawing on Sadri's extensive industry experience, the AI Bootcamp seeks to bridge the gap between academia and AI technology. In this interview, Sadri shares insights into the bootcamp's goals, target audience, and long-term vision.
What is the AI Bootcamp?
The main goal of the EAS AI Bootcamp is to help participants understand when and how to effectively use AI within their research, as well as to identify its appropriate contexts and limitations. Right now, this bootcamp will be a quick but intensive one-week course running from 9 a.m. to 5 p.m., Monday through Friday, and will reoccur eight to 10 times a year. However, we are open to adjusting the frequency and duration based on the feedback we receive.
The bootcamp is geared toward graduate students and postdocs, as these groups stand to gain significant advantages from incorporating AI into their research.
As a comparison, 40 years ago, there were a lot of physicists and chemists who could benefit from using a computer, but they didn't know how. At that time, computers were too expensive and difficult to use. But now, the computer has become a daily tool for everyone. Scientists can benefit from computers quite a bit—running models, doing calculations, performing research, etc. Now, AI is at the stage that the computer was 40 years ago. We must bring scientists on board to be able to use AI effectively.
Who is the ideal candidate for the AI Bootcamp?
The ideal candidate is a graduate student or postdoc who already knows Python and the basic math required to understand AI—linear algebra, calculus, and probability theory. In addition to knowing the underlying science that goes behind machine learning (ML), the ideal participant in the bootcamp should also have relevant research that AI can help with. One goal of the bootcamp is to help participants map their research to fit into a framework where ML works best.
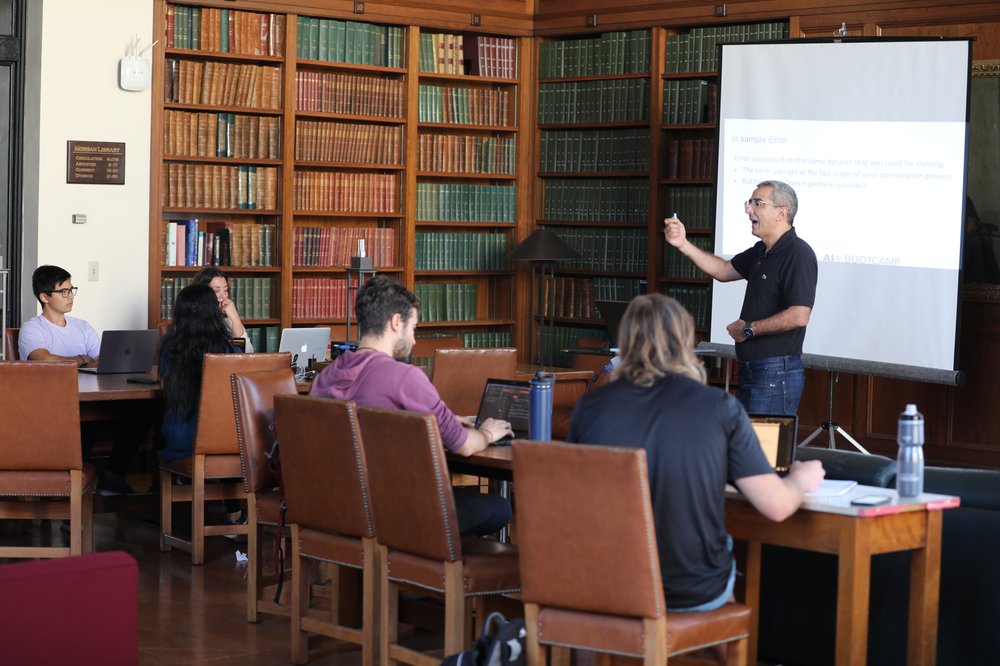
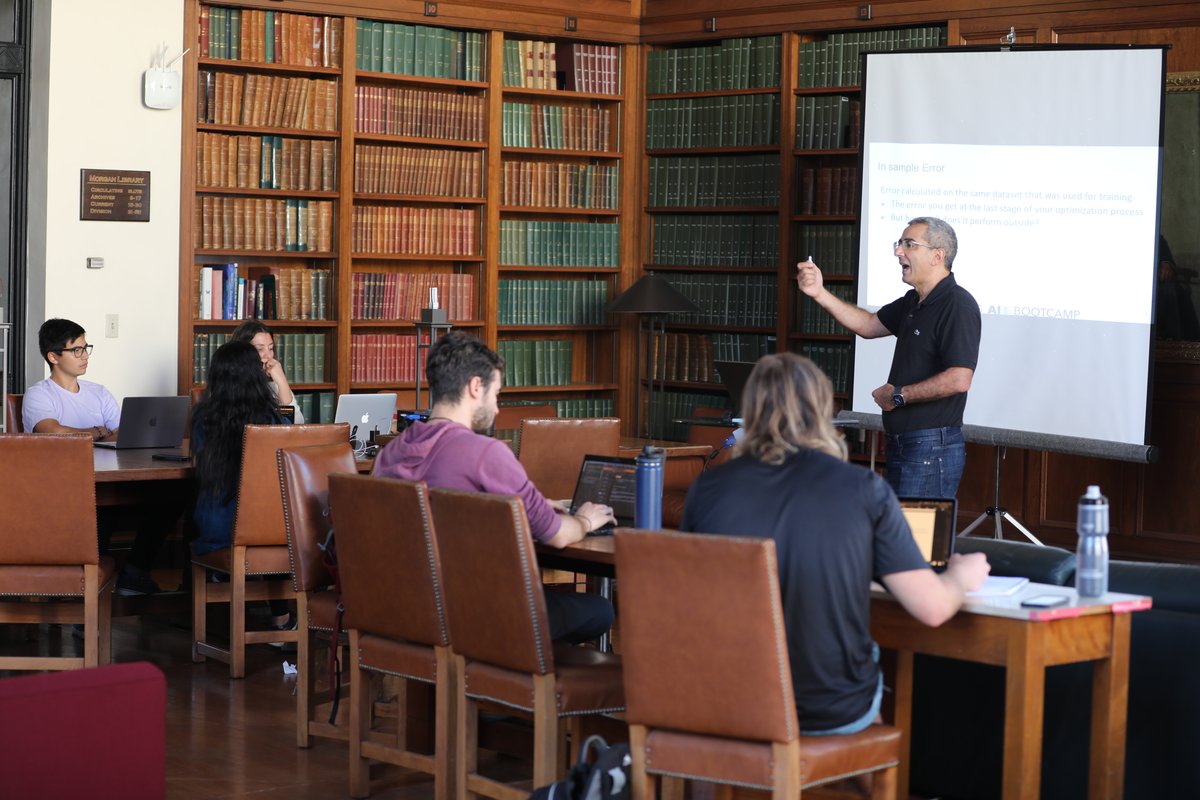
What is the long-term vision for the bootcamp?
The AI Bootcamp focuses on getting people to understand the basics of AI, where to apply it, and where not to apply it. Additionally, the AI Bootcamp will offer sessions focused on using AI for specific domains. In the past decade, AI has expanded significantly into various branches and applications and some AI applications are well suited to specific fields or problems. We will offer specialized bootcamps for these applications, such as reinforcement learning, graph neural networks, and large-scale data processing.
In the first year, our aim is to have about 150–200 participants complete the bootcamp and then gather feedback from them. We plan to establish an ongoing feedback process between the bootcamp participants and the program itself. Using this feedback, we will adjust and make improvements.
We are also considering allowing participants to bring their own research projects and data to the bootcamp. This means if you have a specific project, you can work on it during the bootcamp and utilize any tools provided. However, managing a diverse set of projects and data within the limited time frame is a challenge we'll address in the future.
What is your background in AI, and what led you to Caltech?
My background is mainly in industry, with over 30 years of experience in software development. I initially worked on system software and database management systems. About 13 years ago, I shifted to low-latency data access applications, including financial domains like stock market data processing. This exposed me to AI and ML applications, leading me to develop tools for processing large datasets quickly, applicable in areas like IoT [Internet of Things] and other fields requiring millisecond or microsecond latency.
Then I joined a company called Instacart, where I assembled and managed the team that built the company's machine learning infrastructure. To help build that infrastructure, I collaborated with a diverse group of machine learning engineers who covered everything from search, recommendations, advertisement, and logistics. AI also played a crucial role in economic applications, including incentivizing shoppers, customers, and advertisers.
While at Instacart, I witnessed the extensive use of AI in industry, but I'm intrigued by its wide-ranging applications in the realms of science and technology. Typically, financial incentives drive initial developments, but the diverse applications of AI across various scientific and technological disciplines captured my interest in this position at Caltech.
In addition, I contend that the broader application of AI across diverse scientific disciplines inherently enriches AI and machine learning. This is because scientific research often tackles unconventional problems that are not mainstream, presenting unique challenges. Addressing these issues with AI and machine learning necessitates innovative approaches, thereby pushing the boundaries of the field in unexpected directions.
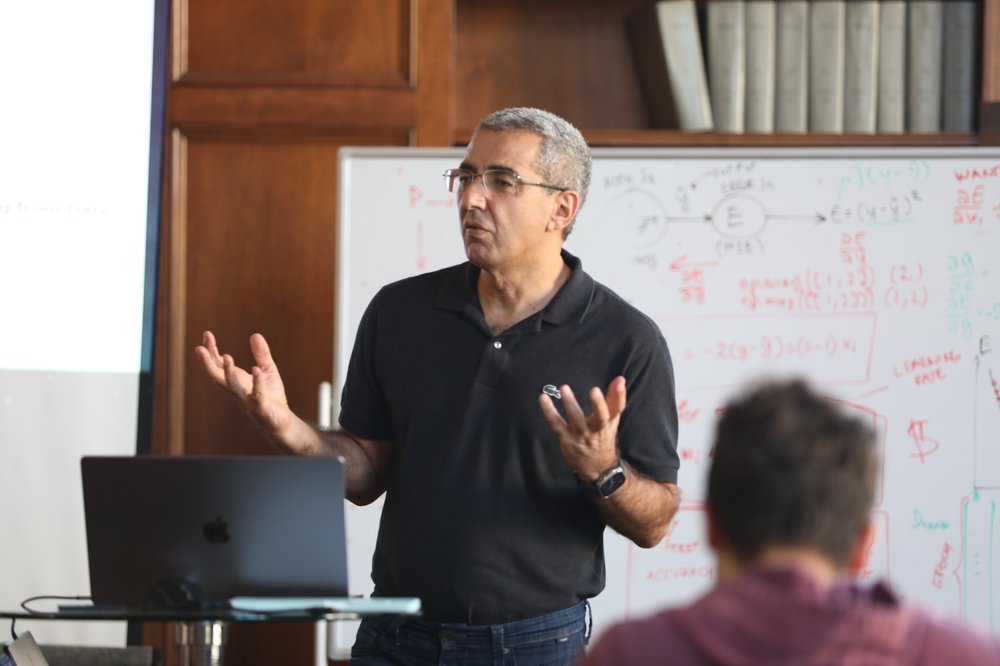
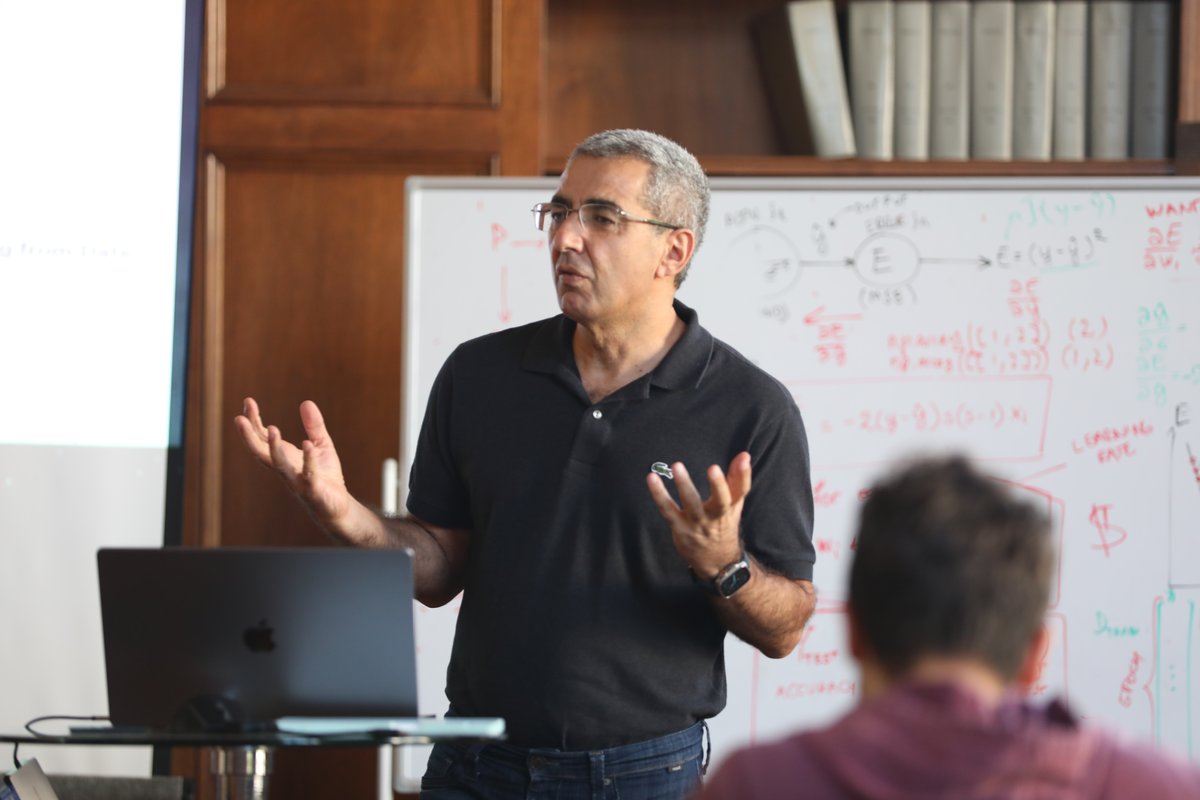
Based on your experience, what do you consider the most common mistakes people make when employing AI in either industrial or research settings?
First, sometimes, you don't need AI. There are some applications where you can get by using simple mathematical models or simple statistics. The second mistake is using the wrong tool. There are many different tools in AI, and some of them are very complicated and complex. Sometimes people use a complicated tool when a simple tool will work just as well. The third mistake is mishandling data. Effective use of data requires clean, relevant, and nonleaky datasets—data leakage is when information from the training dataset is inadvertently included in the model, leading to unrealistic performance. Misusing data like this leads to wasted efforts, such as publishing papers based on incorrect data assumptions.
What are you most looking forward to seeing come out of the AI Bootcamp?
I'm looking forward to seeing significant gains in productivity and satisfaction for researchers across different disciplines of science and technology. While it's challenging to precisely quantify, AI has the potential to improve productivity significantly. Even in basic research tasks, AI simplifies resource discovery. Beyond that, AI aids in experimentation management, with emerging tools such as reinforcement learning for experiment optimization.
AI offers a lot of tools, but understanding of those tools is either nonexistent or superficial. Caltech is full of super smart people, and if I can help the average productivity go up 10 percent, that would be huge.
To learn more about the EAS AI Bootcamp or to get involved, contact Reza Sadri or Melissa Hill.